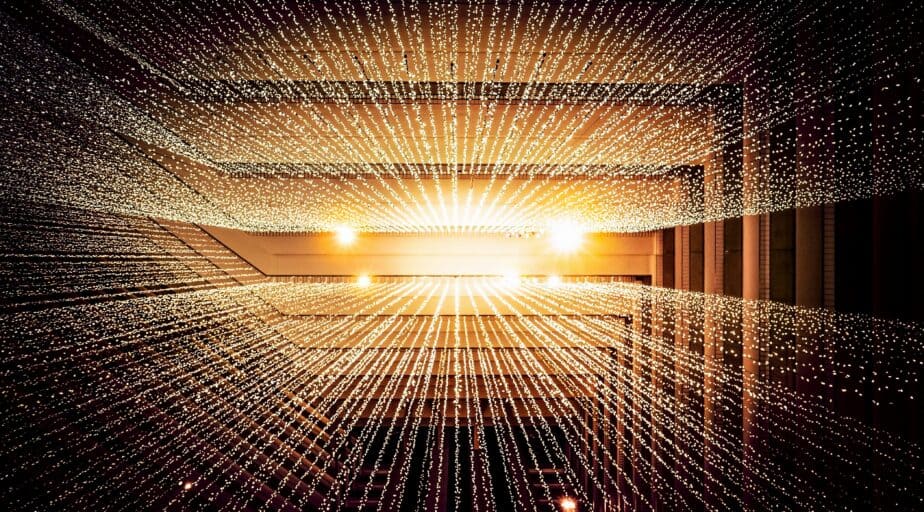
Navigating Data Privacy in the Age of AI: Strategies for Secure Data Analytics
Artificial intelligence (AI) is quickly changing how most of us live our daily lives. From voice assistants to chatbots to Customer Relationship Management (CRM) software, ...
- Datameer, Inc.
- March 4, 2024
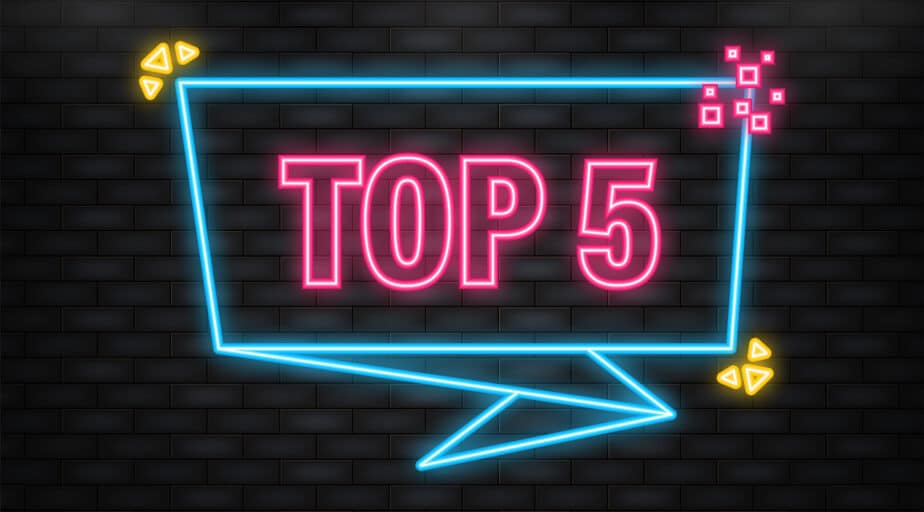
Top 5 Snowflake Tools for Analysts
Explore our expertly curated list of the top 5 Snowflake tools, essential for analysts aiming to harness the full potential of their data with Snowflake’s powerful an...
- Ndz Anthony
- February 26, 2024
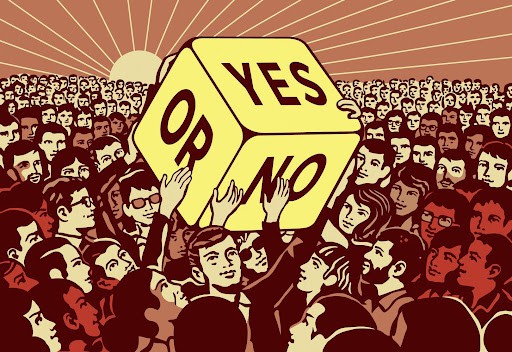
Should You Learn to Code for Data Analytics? – Code vs. No Code
A lot of people automatically associate data analytics with coding. Is this union still ‘legal’ in 2024? We know that the demand for data-driven insights will continue to r...
- Jeffrey Agadumo
- February 20, 2024
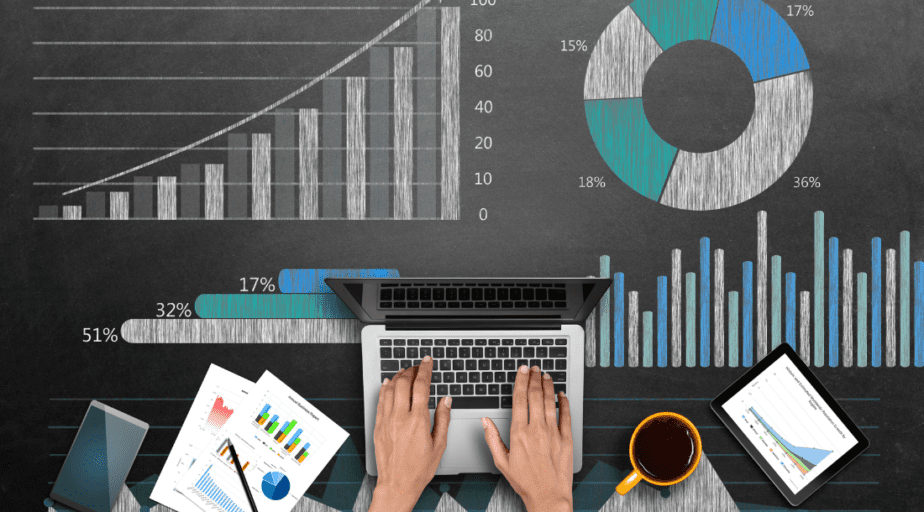
Must Have Data Analysis Tools in 2024
Data analysis tools help to wrangle data – arrange data of different types and in different formats, clean data, and represent aspects of that data to produce actiona...
- Datameer, Inc.
- February 4, 2024
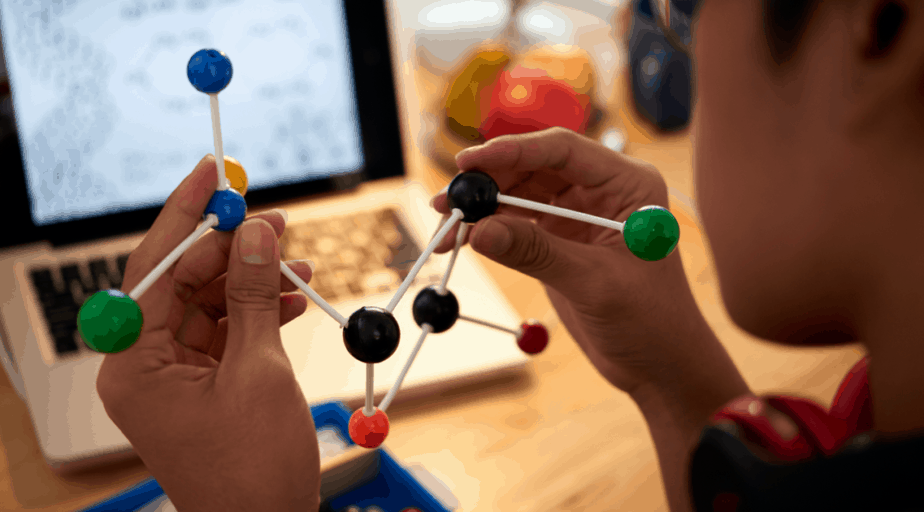
Data Discovery vs Data Exploration: An All-New Look
Much has been made of the term “data discovery.” It is used profusely in the BI market and describes a fundamental transition in BI tools as emphasis has shifted from repor...
- John Morrell
- January 22, 2024
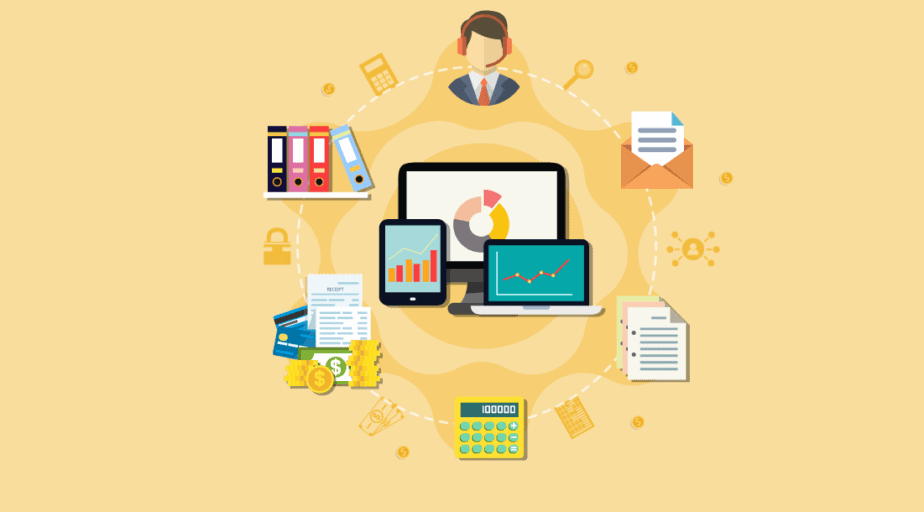
The Ideal Tech Stack for Banks: Optimizing Efficiency and Savings
The banking sector heavily relies on a sophisticated blend of traditional and modern systems. Banks harness various tools ranging from legacy databases like Oracle and IBM ...
- Ndz Anthony
- December 4, 2023
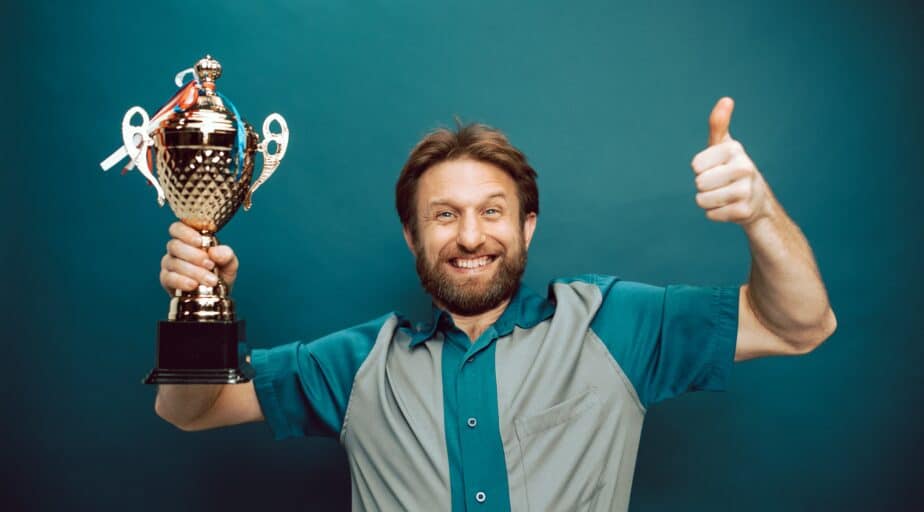
Top 10 World-class Data Transformation Tools for 2024
In an era where data is a critical asset for any organization, keeping up with the latest tools for data transformation is essential. This article presents an updated list ...
- Ndz Anthony
- November 27, 2023
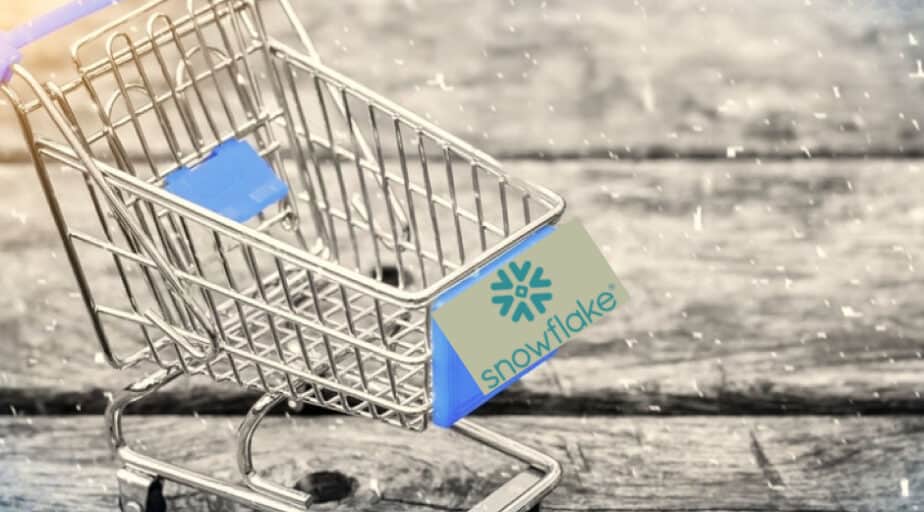
Enhancing Snowflake UI: Part 5 – The Snowflake Marketplace and Its Partners
In the previous parts of this series, we’ve explored various aspects of the Snowflake UI, delving into its features, functionalities, and how to make the most of them...
- Ndz Anthony
- October 19, 2023
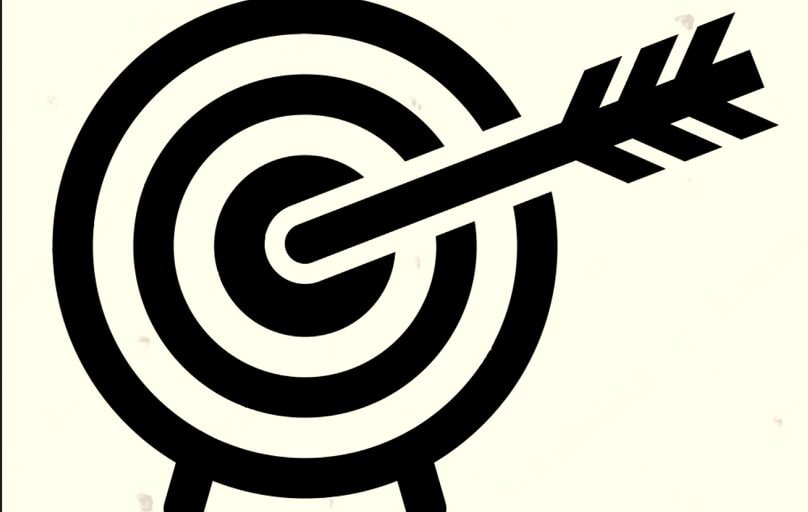
Worksheets Best Practices: Keeping Your Snowflake Worksheets Clean
Did you know that 60% of data analysts spend more time cleaning and organizing data than actually analyzing it? In the bustling world of Snowflake Worksheets, where queries...
- Ndz Anthony
- October 17, 2023
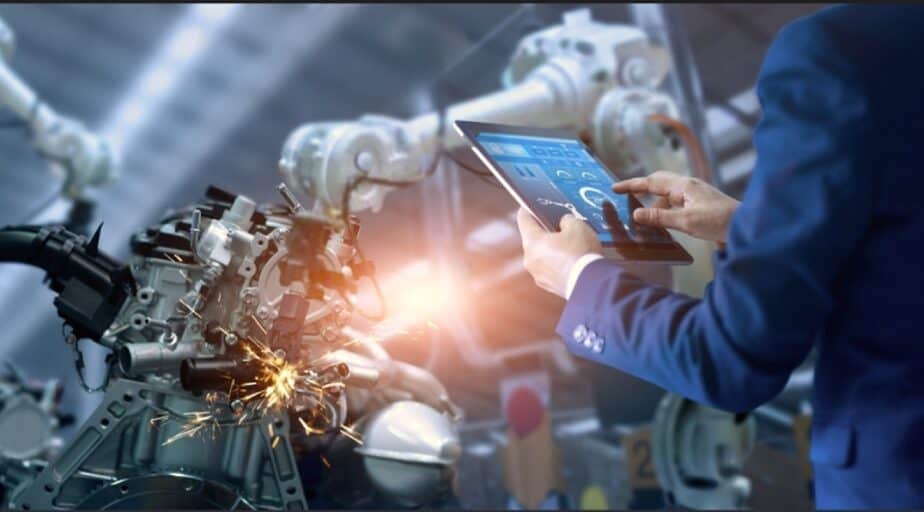
The 7 Most Influential AI Data Innovations in Finance Today
The financial sector has witnessed a significant transformation with the integration of AI. From algorithmic trading to personalized banking, AI’s influence is pervas...
- Ndz Anthony
- October 12, 2023
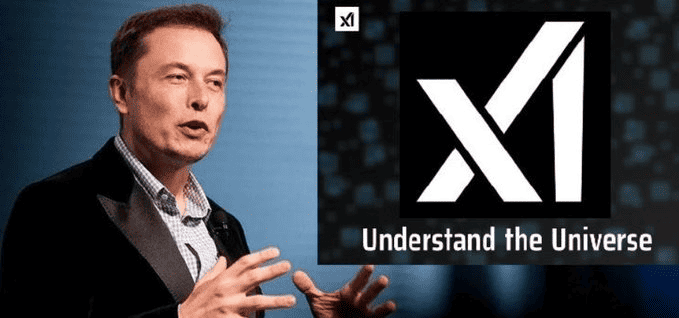
Data Science vs Artificial Intelligence: How Elon Musk’s xAI is Merging the Two Frontiers
Elon Musk’s xAI is at the forefront, merging the frontiers of Data Science and AI in a way that’s electrifying the tech world. It’s not just a technologic...
- Ndz Anthony
- October 10, 2023
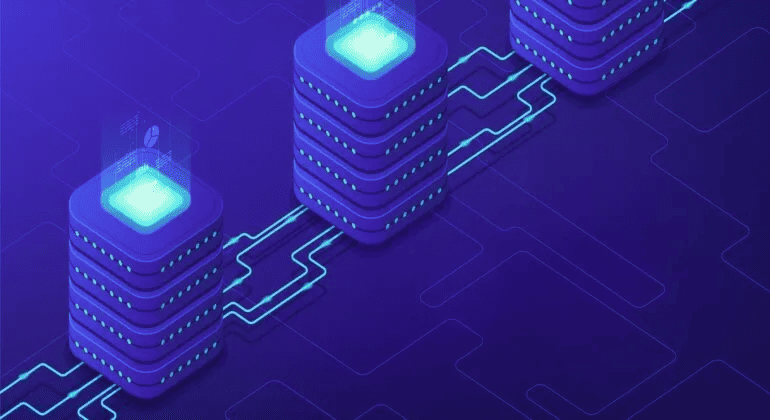
DBT and the Challenges of Large-Scale Data Transformation
As an analyst or data engineer, you’ve just been handed the keys to DBT (Data Build Tool). You’re excited. After all, DBT has been touted as a hero in the realm...
- Ndz Anthony
- October 5, 2023