The Rationale Behind Using Snowflake for Your Data Mesh
- Ndz Anthony
- November 23, 2022
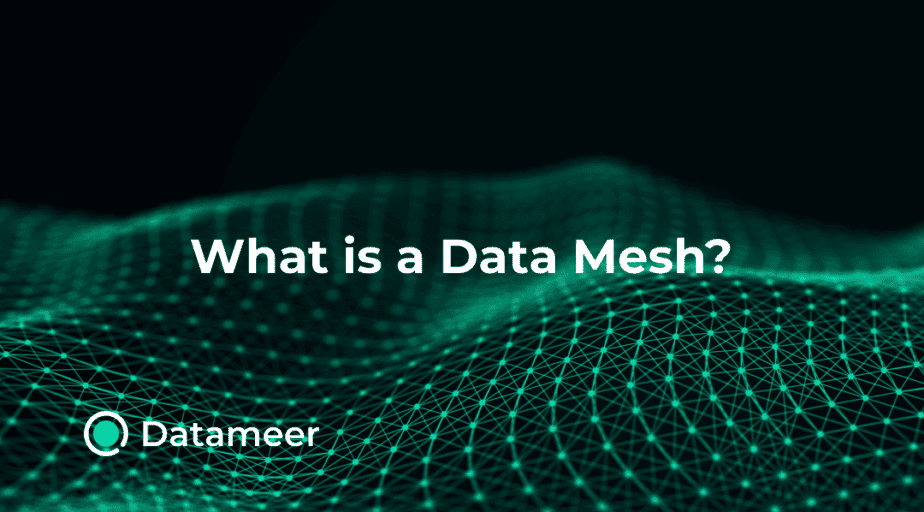
In this article, we will learn how the Snowflake Data Cloud can provide you with the foundation you need to kickstart your Snowflake Data mesh journey.
Most organizations rely on conventional, centralized data architectures, which slow down use-case implementation, obstruct ownership and transparency, and impair data governance.
When the number of data sources and demand for data-use cases increases, the difficulty only worsens.
With data solutions like Snowflake and its data mesh offering, Snow grid, data is decentralized for domain-driven teams.
Follow me through this post to learn how Snowflake Data Cloud provides THE foundation you need for your data mesh journey.
Ready?
Let’s delve in!
What is a Data Mesh?
Zhamak Deghani, director of emerging technologies at Thought works, came up with this phrase.
She referred to this as a method for creating distributed data architectures that support decentralized data management.
The data mesh is not a brand-new paradigm but a fresh perspective on how organizations can make the most of their data architecture to guarantee effective data availability, access, and corporate governance.
What Distinguishes a Data Mesh From Conventional Data Architectures?
Instead of pushing data into a centralized repository, a data mesh’s decentralized structure distributes data ownership.
With a data mesh strategy, domain teams can manage, control, and deliver data as product-aiding accessibility of data throughout the company.
Here’s an image that does justice to this concept.
Let’s take a look at a few salient points:
– A data mesh challenges the traditional idea of consolidating all data onto one big storage platform.
Unlike a conventional architecture where the data belongs to the data teams who manage the centralized repository, data meshing aims to transfer the ownership and power over data to the domain teams.
– Another important distinction is the idea of data products within a data mesh architecture.
According to J. Majchrzak et al., a “data product is an autonomous, read-optimized, standardized data unit containing at least one dataset (Domain Dataset), created for satisfying user needs.”
The domain teams we discussed create and manage their respective data products in a data mesh architecture. This allows the business to realize more value for its customers and the organization.
– Lastly, the mesh strategy aids the management and realization of organizations’ data governance and self-service capabilities.
So what does any of this have to do with Snowflake 🥱?
Let’s find out!
First, What’s Snowflake?
Snowflake is a cloud-based data warehouse. It consolidates data from various sources, allowing you to run in-depth business insights that empower your teams.
Why Use Snowflake For Your Data Mesh?
With Snowflake’s data mesh assistant, Snowgrid eliminates the need for any ETL.
A “Snowflake-backed” data mesh can decrease the time lag between data collection and insights and improve latency for real-time BI activities.
Domain teams can use Snowgrid to securely share a single copy of the data for other domain teams to use asap i.e., all information is current, and any updates are instantly sent to other teams.
And, That’s just the tip of the iceberg.
Here are a few other reasons we think you should consider using Snowflake In Your Data Mesh;
1. Data Governance
It’s a no-brainer that security and access controls should NOT be compromised in favor of a data mesh deployment.
Global governance controls and policies must be considered while creating and disseminating their data products.
With Snowflake’s Snowgrid, the team members’ roles and the data’s sensitivity are considered. Additionally, domain teams can query the views that emerge from datasets in context.
Some note-worthy governance attributes that stand out as it relates to the Snowflake data mesh include:
- Understanding Your Data – Data classification, object tagging.
- Monitoring and Unlocking – Resource monitors to help you monitor your credit usage per virtual warehouse and cloud service.
- Safeguarding Your Data – For this, the Snowflake platform features capabilities like external tokenization, dynamic data masking, and row access policy.
2. Increased Scalability
The Snowflake platform is the backbone of Snowgrid. Snowflake’s robust platform was specifically designed to support scalability, usability, controlled data sharing, and collaboration.
It is well suited to handle centralized and decentralized ownership required for a successful data mesh deployment.
With Snowflake’s underlying technical capabilities, your teams can increase scalability without affecting automation, data quality, or operational performance.
3. Greater Value from Distributed Data
Using centralized target systems can be expensive and frequently results in vendor lock-in.
Consider Amazon Redshift as an example. You pay for each piece of data you send to its warehouse using a pay-as-you-go business model.
Suppose you have large data sets from sources such as CRMs, ERPs, relational databases, platforms, etc. – which as you know, typically gets very expensive over time.
Not with Snowflake!
Snowflake is a platform that allows distributed teams to share data in a controlled and secure manner while remaining connected and avoiding silos.
4. Resilient Computing Capabilities
Snowflake supports separate resources for various teams or workloads. As earlier mentioned, Snowflake provides the skeleton for your data mesh, i.e., You get to leverage powerful features such as Snowflake’s workload isolation, resource avoidance, multiple computing clusters, RBAC governance, etc.
Additionally, Snowflake offers time travel capabilities to help quickly recover your historical data in the event of accidental data loss.
5. Snowflake Data Products For Your Snowflake Data Mesh
From our definition at the beginning of this article.
We have shown that Snowflake is more than just a data warehouse.
In the context of data meshing, Snowflake takes it a step further.
Snowflake allows users to create and serve data products; data, metadata, code, and infrastructure dependencies make up data products in a data mesh.
Here are four categories of Snowflake Data products:
- Code – Task pipelines, stream pipelines, SQL pipelines, Tags, row access policies, etc.
- Metadata – The objects’ names, data types, dependencies, etc.
- Data – External tables, files, views, and functions.
- Dependencies – Pipeline cluster, Task cluster, etc.
Snowflake & Datameer; The Combo For A Sustainable Snowflake Data Mesh
If your organization is planning on transitioning to a data mesh architecture, what better way to begin than with Datameer?
The ‘meer’ in Datameer stands for data mesh…just kidding; not true 😅 — it actually means Ocean.
But what’s true is Datameer’s vision for a unified modeling experience for Snowflake users.
Datameer is a cloud analytics stack built for Snowflake. It serves as a universal semantic layer for your Snowflake data.
With attributes such as SQL visualization, no-code/low-code modeling, data documentation, and ad-hoc analysis, Datameer provides that required level of abstraction your domain and data teams need to collaborate seamlessly.
Looking for a tool to help deploy a quick, adaptable enterprise-wide data mesh strategy?
Click here to preview some of our demos or access a free trial today.