Master Data Management: The Good Cop Policing Your Business Data
- Ndz Anthony
- February 24, 2023
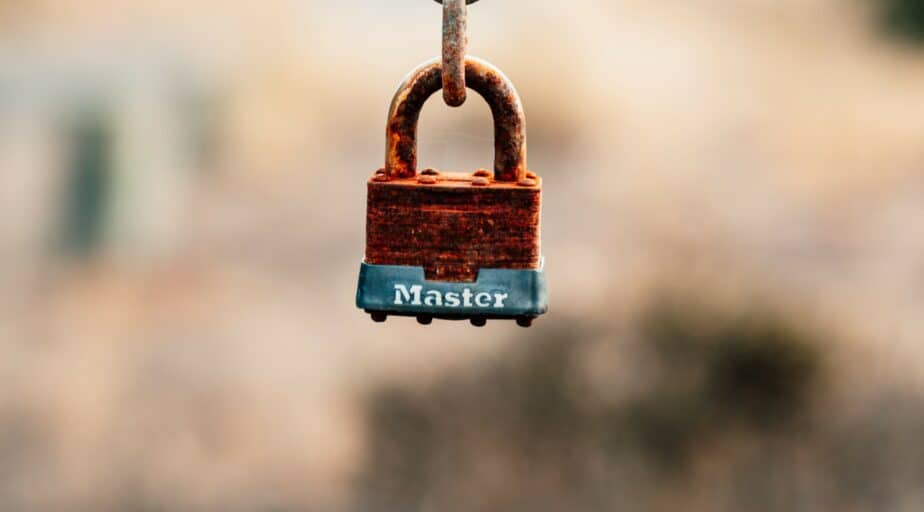
This blog post will explore master data management, benefits, implementation processes, and best practices.
Before we break down the concept of MDM or master data management (for those of us who have a peeve for abbreviations), we need to ask ourselves a very serious question – what exactly are we trying to manage?
Master data, The “MD” in MDM.
Scott Taylor, commonly known as the Data whisperer and one of the early contributors to the master data concept, defines master data as a single source of common business data used across multiple systems, applications, and processes.
He further explains that master data entities can be likened to nouns – the who and the what of your business – entities such as customers, products, vendors, partners, services, etc.
Master, data can be of three types:
1. Reference data: Describes an object’s properties, such as location or product codes.
2. Structural data: Data that explains the connections between various items, such as the connection between customers and their orders, is referred to as structural data.
3. Transactional data: represents interactions between several items, such as sales or inventory data.
What Is Master Data Management (MDM)?
Master data management establishes, maintains, and manages a single, accurate, consistent set of master data across an organization known as master data management (MDM).
It’s the practices and procedures put in place to ensure master data is preserved, maintained, and administered accurately within an organization.
Some examples of 3rd party MDM software are:
- Attaccama
- IBM Infosphere MDM
- Informatica multi-domain MDM
- Master Data Gov with S4HANA
What happens if I don’t implement good MDM practices?
Isn’t it funny how the heading above resembles something out of Quora or Reddit…what happens if I don’t do this (smirks)
I believe it’s a good place to start. One way to do a thing is to know how NOT to do it.
What can happen when an organization does not enforce good master data management?
If an organization does not implement good master data management practices, they tend to face several negative consequences, such as:
-
Data quality issues:
Poor data quality can result in inaccurate, incomplete, or inconsistent information, undermining business decisions and operational effectiveness.
-
Poor operational Efficiency:
Inefficient data management processes can slow down workflows and waste time, reducing productivity and increasing costs.
-
Compliance and regulatory risks:
Failure to comply with data privacy, security, or other regulatory requirements can result in legal and financial penalties.
-
Increased operational costs:
Inefficient data management practices can increase operational costs because data inconsistencies and errors require more time and resources to correct.
Perks of Good Master Data Management(MDM) Practices.
For a company to maintain data consistency and quality, master data management is crucial.
Businesses employ MDM practices to improve data governance, i ntegration , and sharing, amongst other things.
Some of these other advantages include:
1. Data quality and consistency assurance
Master data management aids in ensuring the accuracy and consistency of the data utilized by various systems and processes within an organization.
2. Enhancing data governance
By creating data ownership, governance, and data management rules, master data management enhances data governance.
This guarantees the accuracy and consistency of the data utilized by up and downstream systems and procedures.
3. Enhancing data integration and sharing
By producing a single, precise, consistent collection of master data that can be utilized across many systems and processes, master data management enhances data integration and sharing. This promotes improved decision-making and increases the effectiveness of different corporate processes.
4. Facilitating data analytics and decision making
A good MDM practice provides the backplane for reliable data; With access to a single, reliable, and consistent collection of master data, insights from data analysis can be trusted to provide reliable insights for strategic growth.
5 Steps For MDM Implementation.
Implementing master data management entails several procedures, including data categorization. identification, data integration, data quality,etc.
1. Data identification and classification
Identification and categorization of the key data utilized by various business systems and processes is the first stage in adopting master data management.
2. Data gathering and integration
Gathering and integrating the master data from multiple systems and procedures is the next phase. The data must be extracted, transformed, and loaded into a master data store.
3. Data quality management
The third phase entails executing data profiling and data cleaning to guarantee the quality of the master data. Analyzing the data to find any problems with the data quality, such as missing numbers or discrepancies, is known as data profiling. Data cleaning is correcting errors found during the data profiling process.
4. Data governance and security
Establishing data governance and security rules is the fourth stage in ensuring the correctness, consistency, and security of the master data. Setting up data ownership, data stewardship, and data management policies is part of this.
5. Data maintenance and archiving
The last stage is to maintain and archive the master data. For the master data to remain accurate and consistent, it must be validated and updated regularly. To prevent the master data repository from being overloaded with unneeded data, it also involves archiving obsolete or out-of-date data.
MDM VS Data Warehousing
While there are some commonalities between MDM and data warehouse, they serve different purposes. MDM focuses on managing critical master data entities used across the organization, while the data warehouse consolidates transactional data for analysis and reporting.
However, organizations can benefit from integrating MDM and data warehouses. As a fact, in practice, it is not uncommon for MDM and data warehousing to be included in the same project – to ensure that the data used for analysis is accurate, consistent, and complete.
Wrapping Up With Your MDM + DW Strategy with Datameer.
Hopefully, at the end of this write-up, you now understand the importance of having accurate and consistent data across your organization.
In the previous section, we gave a few examples of 3rd-party MDM tools. In reality, and with many small companies, MDM solutions are not always the best fit. 3rd party MDM solutions are best fitted to larger companies with subsidiaries and disparate systems. For small companies, data warehouses and in-house systems are enough.
With modern DWs like Snowflake, SMEs can automate data governance and management within their Snowflake data warehouse.
With tools like Datameer – a SaaS tool for low-code analytics within Snowflake, one can truly harness the benefits of good MDM practices.
Datameer helps to:
- Standardize data across systems and departments.
- Automate data quality checks and validations.
- Manage metadata and data lineage.
- Provide data governance and security.
- Integrate with other data management tools.
Don’t take my word for it..give Datameer a spin today.