The Role of Data Completeness in Assuring Overall Data Quality
- John Morrell
- August 26, 2021
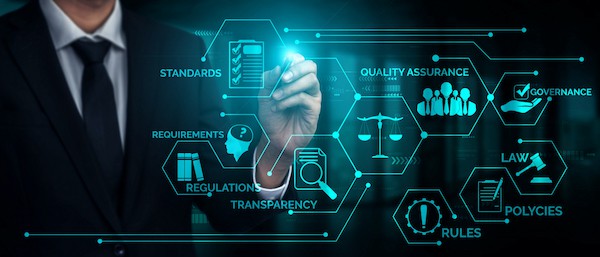
In its early days, the term data quality was often associated with how “clean” or “dirty” a dataset was. Quality was measured by how many erroneous, wrongly formatted, or missing values were in a dataset. A major component of data preparation was data cleansing to improve data quality.
Over time, the definition of data quality expanded to include additional characteristics, including consistency, reliability, and recency (how up to date). When related to data governance, data sets would be labels with “trusted” flags to indicate high degrees of quality, and sometimes data quality scores would be used.
More recently, the terms data completeness and data usefulness have been added to the data quality mix. Many define data completeness as datasets that have no or a limited number of missing values. This points back towards the tasks of data cleansing.
I tend to strive for higher meaning when I describe data completeness. I see data completeness as datasets that have ALL the necessary data to effectively explore and solve an analytical question in-depth, with full context, and at all angles. This definition shines might more on how data sets can improve analytics accuracy and detail. After all, in today’s market economy and microcosms, every organization needs to explore every detail in their analytics to make the proper decisions.
Data completeness is data done right. In this recently released eBook, Maximizing Data Completeness for Highly Effective Decision Making, we explore various methods Datameer customers have used to make their datasets more in-depth and well-rounded to support their decision-making processes. Here is a sneak preview.
Collaboration
The first step in driving greater data completeness is to foster collaboration between your data engineering team and the analytics community (analysts and data scientists). And this does not mean getting them together for drinks on Fridays but rather allowing them to interactively work together on their data pipelines to ensure analytics requirements are met.
Three key data pipeline platform capabilities can contribute greatly to both data usefulness and completeness: reuse, extensibility, and collaboration. Both individually and in concert, these features help DataOps processes increase the production speed, output volume, breadth, and quality of analytics datasets.
Collaboration allows data engineering, analyst, and data science teams to work together to ensure usefulness and completeness. The extended project teams can interactively create, explore, and test data pipelines and datasets to create an agile process that ensures project requirements are met and can adjust requirements as needed.
Data Enrichment
Data enrichment is a highly important yet often overlooked aspect of data pipeline design. It is often overlooked because many data pipeline tools offer limited data enrichment capabilities. Data enrichment features and functions are crucial to gaining high data completeness. Enrichment is also an area where collaboration and extensibility come into play by allowing analysts and data scientists the ability to enrich data in a self-service manner.
A Datameer customer is title insurance, property, and mortgage-related services had highly complex and diverse datasets including data coming from services partners. The diverse data required a heavy dose of coding to normalize, enrich, and classify data for analytics. The customer turned to Datameer to eliminate their dependence on time-consuming, manual SQL coding and took advantage of the rich array of Datameer functions to have data engineering teams normalize and classify data and analysts enrich data to their specific analytics needs.
Data Aggregation & Organization
Making datasets consumable and analytics-ready often requires the data to be materialized into aggregated views or organized in other ways. This allows data to be more easily taken into context and summarized. This is an area often overlooked because most data pipeline tools, as well as for analytics tools, only provide simple means to aggregate data, forcing analysts to write complex SQL.
A leading market research and consumer trends company, and Datameer customer, takes large volumes of consumer purchase and behavior data, organizes and analyzes it, then delivers data and analytics to their hundreds of consumer goods and retail clients. The analytics delivered are diverse with unique requirements. The firm uses the diverse set of Datameer windowing, sessionization, and grouping functions to organize the data, then bucket and aggregate it using intricate dimensions for more effective insights.
Data Science
Most machine learning and AI models require data to be encoded and fed in very specific formats. Very few data pipeline tools offer specific functions to shape and organize your data specifically for data science analytics. Without specific formulas for data science encoding, shaping data for AI and ML can be very tedious and time-consuming
A Datameer customer is the largest multinational pharmaceutical firm in Asia and has high volume, complex, and diverse datasets that feed their data science projects and operational models. The data science projects require wide and deep datasets that have enriched and encoded columns specific to the model.
Prior to using Datameer, the data was blended, enriched, and encoded by coding within their data science notebooks – a time-consuming and error-prone process with limited reuse and operationalization. With Datameer, the firm is able to organize, enrich, and encode the data within their data pipelines in a fraction of the time without coding using the rich set of Datameer functions.
Wrap Up
These a just a few examples of how Datameer customers are able to maximize data completeness, make datasets more useful to their analytics community, and drive highly effective decision making. One key to the success of customers such as Citibank, Royal Bank of Canada, British Telecom, Bank of Montreal, Aetna, Optum, Morningstar, Vivint, and more is having a collaborative data transformation tool.
Datameer SaaS Data Transformation is the industry’s first collaborative, multi-persona data transformation platform integrated into Snowflake. The multi-persona UI, with no-code, low-code, and code (SQL) tools, brings together your entire team – data engineers, analytics engineers, analysts, and data scientists – on a single platform to collaboratively transform and model data. Catalog-like data documentation and knowledge sharing facilitate trust in the data and crowd-sourced data governance. Direct integration into Snowflake keeps data secure and lowers costs by leveraging Snowflake’s scalable compute and storage.