Data Preparation for Machine Learning: A Complete Guide with Datameer
- Ndz Anthony
- August 29, 2023
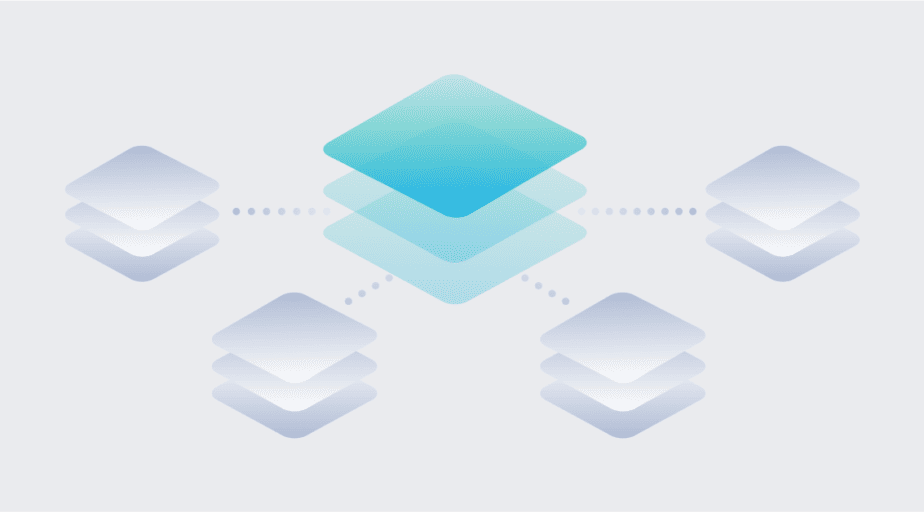
Data is the fuel for machine learning. Without data, there is no machine learning. However, not all data is created equal. In fact, most of the data that we encounter in the real world is messy, incomplete, inconsistent, noisy, or irrelevant.
This makes it hard or even impossible for machine learning algorithms and models to learn from it and produce accurate and reliable results.
And that’s why data preparation is a crucial and often overlooked step in any machine learning project.
You could rush ahead and feed this raw data into your ML algorithm, hoping for the best. But as many businesses have learned the hard way, this approach often leads to biased results and limited scalability.
Take Spotify, for instance. When developing their recommendation engine, they faced significant challenges related to data quality. It was only after investing considerable effort in cleaning, normalizing, and transforming their data that they were able to train an ML engine capable of delivering highly personalized music recommendations.
This example underscores a crucial truth in machine learning: the success of your ML model hinges on the quality of your data. And since all datasets are inherently flawed, data preparation is a non-negotiable step in the machine learning process.
This post provides you with a complete guide on how to use Datameer {one of the most painless ways} to prepare your data for machine learning. We will cover the following topics:
- Data Cleaning
- Data Transformation
- Data Preprocessing
- Feature Engineering
But before we get into the business of the day, we can have a glimpse of what data preparation is all about.
Data For Machine Learning: What is Data Preparation?
Data preparation is the process of transforming raw data into a format that is more amenable to modeling. It’s a critical step in the machine learning pipeline, bridging the gap between data collection and model training.
While the specific tasks involved in data preparation can vary depending on the nature of the data and the problem at hand, there are several common tasks that often come into play. These include:
- Data Cleaning: This involves identifying and rectifying errors in the data. It could be anything from dealing with missing values and outliers to correcting inconsistencies in the data.
- Feature Selection: Not all data is created equal. Some variables are more relevant to the task at hand than others. Feature selection involves identifying these important variables and focusing on them.
- Data Transforms: Sometimes, the raw data isn’t in a format that’s ideal for machine learning. Data transforms involve changing the scale or distribution of variables to make them more suitable for modeling.
- Feature Engineering: This involves creating new variables from the existing data. These new features can often provide new insights and improve the performance of the model.
- Dimensionality Reduction: High-dimensional data can be challenging to work with. Dimensionality reduction techniques create compact projections of the data, reducing the number of dimensions while preserving the essential structure of the data.
These tasks provide a general framework for data preparation. However, it’s important to remember that the specific steps and techniques used will depend on the data you’re working with and the problem you’re trying to solve.
In the next section, we’ll take you through how you can perform these data preparation tasks using Datameer.
How to Prepare Data For Machine Learning Using Datameer
Datameer offers a robust platform for performing data preparation. For simplicity and clarity, we’ll divide the process into three main steps: Selecting Data, Preprocessing Data, and Transforming Data.
Step 1: Selecting Data
The first step in data preparation is selecting the data that will be used for machine learning. This involves identifying and gathering the relevant data from your various data sources. With Datameer, you can easily connect to a wide range of data sources, from databases and data warehouses(like Snowflake) to cloud storage and web services.
Once you’ve connected to your data sources, you can use Datameer’s intuitive interface to explore your data and select the relevant datasets. You can also use Datameer’s powerful search and filter capabilities to quickly find the data you need.
Step 2: Preprocessing Data
Once you have your data in place, the next station is preprocessing. Here, you’ll clean the data and tackle issues such as missing values and outliers. Datameer equips you with a variety of tools for data cleaning, including functions for managing missing data, identifying and eliminating duplicates, and addressing outliers.
Preprocessing isn’t just about cleaning; it’s also about feature selection. You need to identify the variables that hold the most relevance for your machine learning task. Datameer’s analytics functions can assist you in analyzing your data and spotlighting the most crucial features.
Step 3: Transforming Data
The final leg of the data preparation journey is data transformation. This step is all about tweaking the scale or distribution of your variables to make them more amenable to machine learning. You might need to normalize your data or convert categorical variables into numerical ones.
Datameer offers a broad spectrum of data transformation functions. Whether you need simple operations like addition and multiplication or more complex functions like logarithms and exponentials, Datameer has got you covered. You can even use Datameer’s formula builder to craft custom transformations.
Always remember, when you dedicate significant time and energy engineering features from your dataset, it can significantly enhance the algorithm’s performance. Adopt an incremental strategy: start small and use the knowledge you gain to continue building your skills.
I hope this blog has inspired you to try out Datameer for your own machine learning projects.