4 Game-Changing Strategies to Maximize Smaller Analyst Teams
- Jeffrey Agadumo
- January 27, 2023
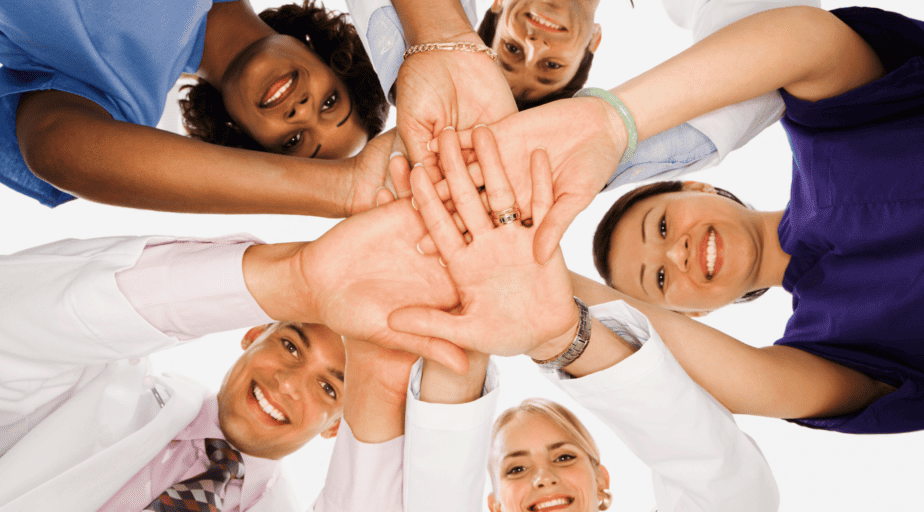
What do you do when you have a small analyst team? This article provides 4 proven productivity strategies to maximize the effectiveness of your smaller analyst team.
Real-time data within organizations involve complex data sets that usually contain thousands of features and many more observations.
Hence it could be challenging to figure out what data model to build and what tools to use in processing your model. The scope may be too wide for one individual to handle.
To segment data projects into more manageable workloads, you would require a larger team of data specialists with different expertise and an extensive understanding of your organization’s data.
A Data-Driven World
About 4.9 billion people are reportedly using the internet today. And these people generate over 2.5 quintillion bytes of data daily – that’s 18 zeros, in case you were wondering.
With such a large pool, intelligent businesses rely on data insights to make decisions regarding customer acquisition, retention, and product design.
To effectively utilize data, it is necessary to have a team of analysts who can maximize data efficiently for better insights.
But, finding and maintaining a robust team of analysts with a broad range of skills is not always the easiest thing for an organization. And here are some reasons why:
- Demand for Data analysts with a broad range of skills is highly sought after, this drives up salaries making it more difficult to find and hire top talent.
- Organizations may need to invest in upskilling their data analysts to stay up-to-date with the latest tools and techniques, this makes the cost of training and development very high.
- Data analysts with a broad range of skills may require more complex and expensive equipment and software to work effectively, which can also add to the cost of maintaining a team.
Have a Smaller Analyst Team? Make The Most of Them
Overall, the high demand for talent and the need for upskilling can make finding and maintaining a solid team of skilled data analysts expensive.
However, there are methods that small analyst teams can utilize to enhance their productivity and achieve the best results on each project.
The following points will help you make the most out of your smaller data analyst team:
1. Setup a Modern Data Stack
Selecting the right tools remains the most essential consideration in your ETL process . Failure to do so ensures a longer turnaround time for data processing.
And that is why switching to the modern data stack should be at the top of your list.
The Modern data stack describes tools and technologies that give a seamless and efficient flow during data integration.
It is typically cloud-based, centralized, and modular. They are designed to support a wide range of data-related activities like data warehousing, integration, visualization, and machine learning.
Your data stack should include relatively fast, easy-to-use tools and no-code options to reduce the learning curve for junior team members.
Typically you would require tools to handle the following tasks in your stack.
- Data Collection: This is gathering data for business decision-making, strategic planning, research, and other purposes.
- Data Warehousing: Integrates data and information collected from various sources into one comprehensive database.
- Data Transformation: Cleaning and sorting data (usually in different formats) to have a uniform structure.
- Business Intelligence (analysis): Creating a model for analyzing the data, visualizing the results, and developing insights from them.
2. Diversify Your In-House Skill Set
Even within a small team, teammates should, as the saying goes, “know their onions.”
They must understand how to use the tools in the team’s data stack.
They also have to be skilled in one or more programming languages for data analysis, like SQL or Python (no-code alternatives like Datameer work great in this instance too).
To make a small data analyst teamwork, your analytics team should have the following roles on board:
- Data Engineer: to build and maintain the systems and architecture that allow data scientists to access and interpret data.
- Data Analyst: analyzes existing data across the business to derive key insights for making strategic business decisions.
- Data Scientist: to create new ways of capturing and analyzing data for analysts to utilize, they develop the tools a data analyst will use.
- Analytics Engineer: a newer role gaining more and more notoriety, they provide clean, accurate datasets that different users within the company can work with.
3. Automate Your Workflow
Businesses often use task automation software to simplify and speed up workflows. This alleviates burnout and fatigue from repeatedly doing the same task.
For instance, data preparation is one of the most time-consuming but important stages in the ETL process. If you save time in this process, your team can focus on more important tasks, like interpreting data and developing valuable insights.
Most task automation software helps you automate data analytics, pipeline, and engineering processes.
And data warehouses like Snowflake offer automated and scalable pipelines to accelerate your data preparation and other processes.
4. Avoid Analysis Paralysis
When companies often set out on their data analytics journey, they want to dip their toes in as much data as possible.
But how much data is too much data?
Given the time it takes to organize data and generate insights, analysts and even entire teams can become frozen in indecision or hooked on gathering more data.
This is commonly known as analysis paralysis. And here are some tips on how your team can avoid this:
- Accept that uncertainty is inevitable . Maintain your effort and still be thorough and detailed, but remember that uncertainty is inevitable in any analysis.
- Have better data accessibility . Easy access to a centralized data warehouse will allow a more comprehensive analysis to be done quickly.
- Always keep your objective in mind . Keep your initial goal in mind when getting conclusions and insights and follow standard data practices.
Generally, with the endless amount of information available, it’s easy to get lost in the numbers.
There will always be additional factors that give room for further evaluation when analyzing.
However, it’s vital to consider the factor of diminishing returns when investing time into analyzing more data. Remember! Time is money.
One final trick! Datameer
Handling data storage and transformation without changing tools too often is considered by many data analysts to be the ideal option. That seamless integration cuts down the turnaround time by a lot.
And Datameer offers you just that! With Datameer’s no code, low code, and SQL options in data transformation, team members with different skill sets can collaborate seamlessly.
What does this mean for your small team of data analysts?
Superpowers!
Your small team will go from long hours and using different tools to less time spent on each project without compromising on the quality of your data insights.
Ready to enter hero mode with your team?
Click here to find out more about what your small team can achieve!