How Is Tableau Used for Data Science?
- Justin Reynolds
- January 2, 2020
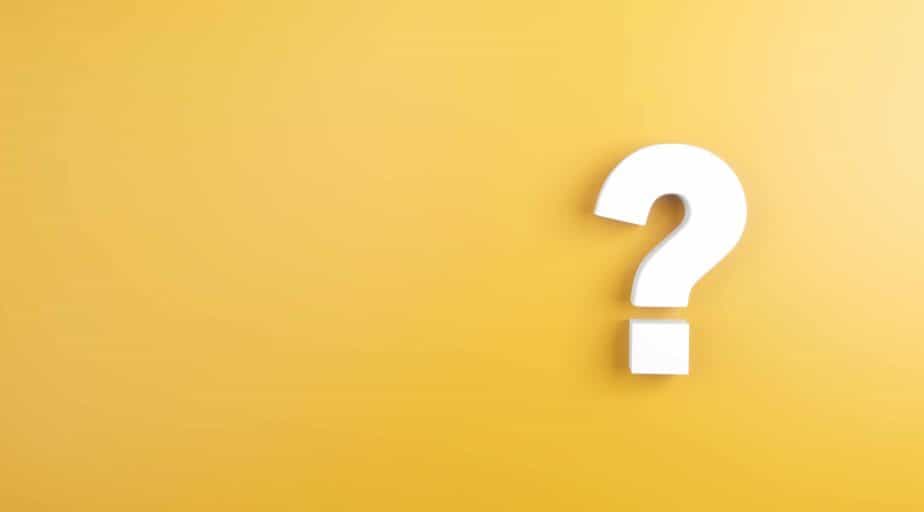
Organizations today collect massive amounts of data to make informed decisions and discoveries—and to edge out competitors. Yet the majority are still struggling to extract meaningful insight from the structured and unstructured data they’re harboring.
In a recent study, 53 percent of respondents said their team lacks the IT skills or staff to extract useful insight from data . What’s more, 50 percent lack the tools to feed downstream apps with timely and relevant information, and 44 percent don’t have the time to sort through data.
At the same time, 31 percent of respondents said their organization hasn’t invested enough in platforms that streamline data analytics .
Add it all up, and these numbers highlight the need for services like Tableau, a cutting-edge platform for data visualization and business intelligence.
Tableau was acquired by Salesforce in June 2019 for $15.7 billion, making it the largest acquisition by Salesforce to date. Now fully integrated with Salesforce, Tableau is the clear market leader in data visualization software and a must-have tool for any data science team.
What is Tableau?
Tableau is a platform for discovering, preparing, visualizing, and sharing data. It’s highly scalable and is used by data science teams across all vertical markets.
There are four main products within the Tableau product family , including Tableau Prep for preparing data, Tableau Desktop for delivering insights across the enterprise, and Tableau Server/Online for hosting and sharing data.
The company also offers add-ons (e.g., Tableau Data Management and Server Management), embedded analytics, and developer tools like webhooks for advanced integrations, customizations, and automation.
How Tableau is Used for Data Science
Despite its popularity, Tableau isn’t the only tool that data scientists are using today. Data science is a massive field with many areas, so analytics teams often use several tools together for data science purposes.
For example, Tableau can be used in conjunction with programs like MatLab for predictive analytics, Alteryx for data blending, Datameer Spotlight for finding, accessing, and combining data sources, and Anaconda for data discovery and analysis, among many other tools.
Still, because communication is critical to the success of analytics projects, Tableau remains an incredibly important tool for data science teams.
Data scientists often need to break down information and make it digestible for internal teams, C-level executives, colleagues, and customers. As such, data must be presented in a way that’s easy to view and understand—which is where Tableau shines.
Using Tableau, analytics teams can drill down deep into data, uncover hidden insights, and present the findings in a way that tells a highly visual, compelling story. Tableau is great for quickly exploring data, slicing and dicing it, and packaging it in a way that is interactive, collaborative, and visually pleasing.
Another reason why Tableau is so popular is that it can connect to a wide variety of data sources. For example, teams can connect Tableau to cloud systems like Azure or Google BigQuery, relational systems like SQL Server and Oracle, and file systems like Excel and CSV, among others.
Tableau as a Data Science Enabler
Using Tableau improves the way analytics teams comprehend and present data, in turn strengthening collective data scientist skill sets. It’s also excellent for quickly pulling together reports when they are needed—without having to manually build visualizations.
Tableau is not ideal for every single data project; some programmers will prefer to use tools like ggplot2 (for R) or the open source pandas library (for Python), for example. These tools offer a bit more flexibility. But, like anything else, they also come with some drawbacks.
“Sometimes you may not know or remember the code to present data a certain way,” Jason Widjaja, global data science associate director at MSD, recently wrote on Quora . “Sometimes the data format is tricky but Tableau has a connector. And Tableau is a leading tool. Not particularly in terms of functionality, but in terms of its broad distribution, so there is a comparatively higher chance you will encounter it.”
Ultimately, it’s important to keep learning and exploring new ways of visualizing data.
“Tableau itself is a tool. And only a tool,” Widjaja continues. “It is primarily doing data visualization well that helps you become a better data scientist—not learning a particular data visualization tool, Tableau or otherwise. Data visualization helps you explore the data to ascertain its underlying structure, communicate your findings in an intuitively understandable way using our brains inbuilt pre-processing capability that kicks in long before any conscious thought occurs, and helps you sell the ideas that will fund your data science and analytics unit.”
Get Started Using Tableau
Getting started with Tableau is not as hard as it might sound—even for beginners. The platform offers a robust learning community with free training videos, live training sessions, and certification programs. Head over to their website to access Tableau’s learning resources .
In addition, Tableau provides free access to Tableau Prep Builder and Tableau Desktop for students and instructors around the world, making it easy to compile data for lectures, presentations, and thesis projects. Explore Tableau’s academic programs .
Data Transformation Remains a Challenge
The introduction of Tableau Prep provides a slightly more flexible and automated way to prepare your data – blend and transform – for analytics in Tableau. But Tableau Prep has major limitations as you can see in our comparison guide of Datameer and Tableau Prep, particularly for data science datasets.
Datameer SaaS Data Transformation is the industry’s first collaborative, multi-persona data transformation platform integrated into Snowflake. The multi-persona UI, with no-code, low-code, and code (SQL) tools, brings together your entire team – data engineers, analytics engineers, analysts, and data scientists – on a single platform to collaboratively transform and model data. Catalog-like data documentation and knowledge sharing facilitate trust in the data and crowd-sourced data governance. Direct integration into Snowflake keeps data secure and lowers costs by leveraging Snowflake’s scalable compute and storage.
Are you looking for a no-code or low-code data transformation solution for Tableau that your data and analytics team can use?
Learn more about our innovative SaaS data transformation solution with direct integration into Snowflake, Sign up for your free trial today!