Organizing Analytics Teams for Success
- John Morrell
- December 2, 2019
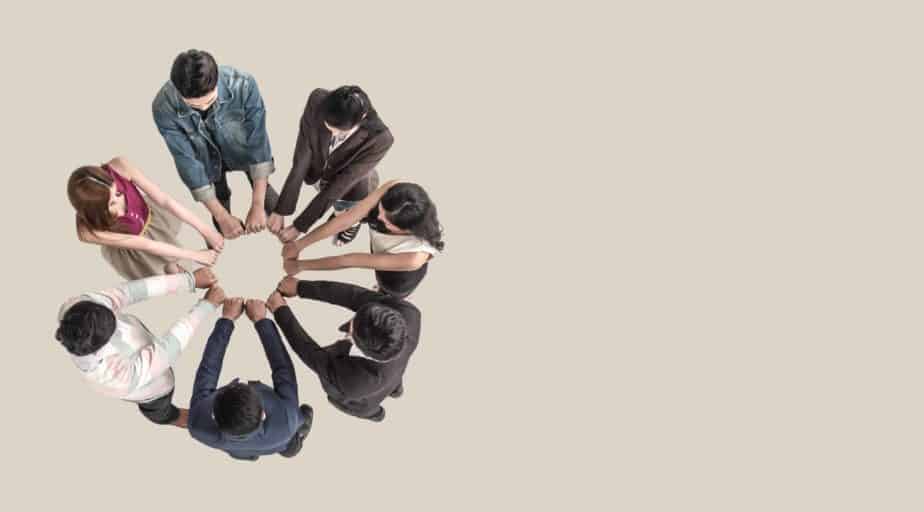
There are different models by which a company can organize analytics teams for success. Often time this model reflects the overall structure, operating model, and culture of the company. The key is that it blends in a way to successfully serve the business and drive effective execution.
No one analytics team organization model is necessarily better than the other. At Datameer Spotlight, we stress the need for collaboration and knowledge sharing as a critical factor to successful analytics programs, regardless of how it is organized. Let’s take a look at different organizational models.
Organization Factors
There are three general ways companies can organize analytics teams for success: Centralized, Decentralized, and Mixed. Each of these can also have different approaches in how they best serve the business based on the operating model and culture of the company, creating multiple models within each general approach.
There are also different operational and cultural factors taken into account when determining the right model, which includes:
- How is the overall business structured? Are there more centralized management teams, autonomously operating business units, or a mix?
- How are decisions made and executed? Is decision-making centralized and top down, or are teams autonomous and have their own authority?
- What is the culture of the company? Are managers and knowledge workers trained and encouraged to think independently and to
Let’s examine each of the three models the secrets to making them successful.
Centralized
In this model, there is a central analytics team that serves different functional groups and business units. The team will typically have multiple roles – data architects, data engineers, data analysts, data scientists, and project managers – with a diverse set of skills.
One way for the centralized model to operate is for different functions and business units to submit projects to the team. The analytics team will then scope the projects, build requirements, assign team members and execute.
A slight alternative is for the central analytics team to take a more consultative role. The analytics unit would create an analytics strategy, consult across the organization, and execute on broader initiatives that the functions and business units would fund.
Two key success factors to the centralized model are having a well-communicated organization-wide analytics strategy and a series of best practices to deliver on that strategy. Both play an integral role in explaining and executing analytic initiatives across the company.
Decentralized
In a decentralized model, analysts (data and business) are typically deployed out in the organization in specific lines of business and/or departmental functions (marketing, sales, etc.). “Data management specific” roles (data architects and data engineers) may remain centralized to effectively manage the flow of data across the organization and be applied to specific analytics initiatives across the business teams.
There are typically two key tasks of the analysts in the business: (a) continuously analyze and monitor the various performance metrics of the unit, and (b) answer new questions that arise in the business, often on a daily basis. The former task is typically done via structured projects that deploy a regimented flow of data, while the latter requires analytics teams to discover and explore new analytics assets, and collaborate with team members to deliver new analytic results.
Critical success factors to the decentralized model are communication, collaboration, and sharing. With the analytics community spread throughout the organization, analysts need to know about various analytics initiatives and the assets that are created to accelerate their own initiatives and help keep consistency in how the organization produces analytics results.
Mixed
In a mixed analytics organizational model, there may be some analytics teams that are centralized performing cross-departmental analytics, while others may be deployed into the business. As with the decentralized model, data management functions are likely centralized to manage the flow of data.
For example, there may be a central team that manages all performance metrics analytics across the organization, while decentralized teams help answer deeper analytics questions that arise in the various units/functions. Using our example from the decentralized model, the central team might deliver analytics that produces the standardized performance metrics via structured projects, while the local teams would answer new emerging questions in an ad-hoc manner for the units/functions.
As with the decentralized model, communication, collaboration and sharing are key. Even in this mixed model, teams are distributed and need complete communication and sharing around initiatives, various analytics standards, and different analytics assets that are available across the organization.
The Center of Excellence: A Common Thread
Successful organizations have found that a Center of Excellence (COE) plays an important role in creating an effective analytics program. In each of the three models we discussed, a COE facilitates the success factors:
- In the centralized model, the COE helps create the organization-wide analytics strategy, builds a blueprint, creates a series of best practices, and plays an integral role in explaining analytics to the rest of the company.
- In the decentralized model, the COE helps facilitate the overall strategy, creates the best practices, put technology standards in place, and facilitates the communication and sharing required.
- In the mixed model, the COE performs similar tasks from the decentralized model, but also sets wider standards in and around how organization-wide metrics are calculated, and also facilitates standards and knowledge around data.
The COE will typically be under the purview of the VP of Analytics, Chief Analytics Officer, or Chief Data Officer, depending on which title and functions the organization decides to deploy.
Collaborative Data Transformation is Critical
Regardless of how your teams are organized, it is critical to foster collaboration among the data and analytics teams. Oftentimes data teams know a great deal about how data is managed and structured, while analytics teams know a lot about the business uses the data. The two teams need to mind-meld and share their two knowledge sets for fast, successful analytics projects.
Datameer is a powerful SaaS data transformation platform that runs in Snowflake – your modern, scalable cloud data warehouse – that combines to provide a highly scalable and flexible environment to transform your data into meaningful analytics. With Datameer, you can:
- Allow your non-technical analytics team members to work with your complex data without the need to write code using Datameer’s no-code and low-code data transformation interfaces,
- Collaborate amongst technical and non-technical team members to build data models and the data transformation flows to fulfill these models, each using their skills and knowledge
- Fully enrich analytics datasets to add even more flavor to your analysis using the diverse array of graphical formulas and functions,
- Generate rich documentation and add user-supplied attributes, comments, tags, and more to share searchable knowledge about your data across the entire analytics community,
- Use the catalog-like documentation features to crowd-source your data governance processes for greater data democratization and data literacy,
- Maintain full audit trails of how data is transformed and used by the community to further enable your governance and compliance processes,
- Deploy and execute data transformation models directly in Snowflake to gain the scalability your need over your large volumes of data while keeping compute and storage costs low.
Learn more about our innovative SaaS data transformation solution, Sign up for your free trial today!