Four Ways Big Data Helps Deliver Value from AI
- John Morrell
- February 27, 2018
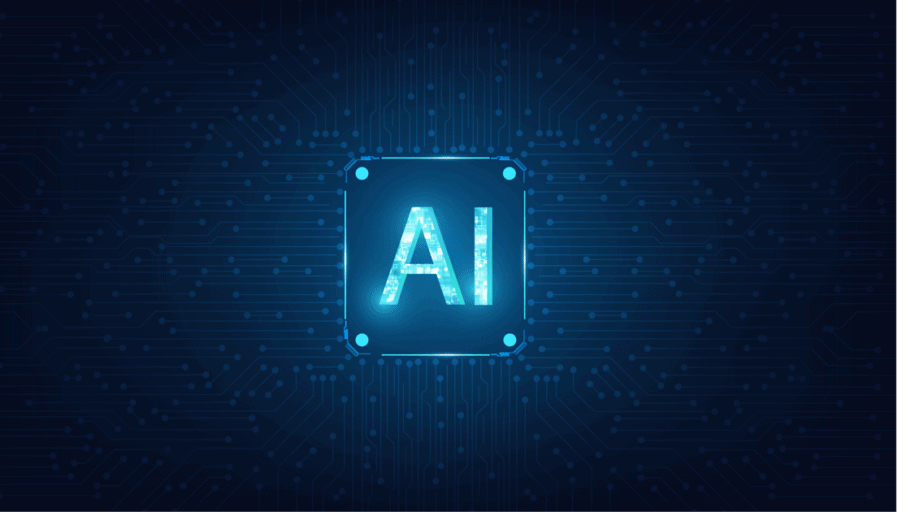
Where Has AI Struggled?
Because AI has been mostly in the realm of data scientists, who tend to be more technically savvy than their business analyst counterparts, little focus has been paid to the operationalization aspects:
- How do I get AI embedded in my analytic workflows?
- How do I get analysts to use more AI to get insights?
- How do I get IT to bless the use of AI in production environments?
With no sense of urgency to get results to the business, little effort was placed on shortening delivery cycles.
Historically, AI projects were continually plagued with complex analytic cycles. Data scientists spend 80+ percent of their time doing non-data scientist stuff, in particular, complex data engineering tasks and writing code to re-implement models.
BI and analytics teams are the ones responsible for creating analytic data flows that the business uses every day. Little democratization of data science has occurred, often times because the data scientists and their unique tools are off in their own world, disconnected from the main business analyst teams. This created little synergy between AI models being created and real-world business processes the analysts are trying to improve.
Big Data and AI: A Marriage Made in Heaven
Two emerging factors are advancing the cause of AI for enterprise analytics:
- New convolutional neural networks are making it easier than ever to create deep learning models that can be rapidly applied to business problems
- Enterprise data engineering platforms give data scientists the tools they need to quickly create analytic pipelines that can feed more data to new AI models
Each of these on their own speeds the AI analytic delivery cycle. Combining the two is a situation where 1+1=3, because they also address the data science democratization and operationalization challenges that plague AI today.
Modern big data platforms have dramatically changed the landscape entirely, upping the ante in terms of data size, faster time to insight and analyst efficiency to create next generation analytics to drive the business.
Connecting AI to these modern big data platforms would help deliver these same benefits to AI-enriched insights and democratize the use of AI in many parts of the organization. Let’s look at four ways AI can benefit from integration with big data platforms:
1. Faster Cycles
Disjointed analytic cycles and non-optimal tools slow down the delivery of AI-enriched insights. The marriage of big data engineering platforms such as Datameer, with next-generation deep learning platforms such as Google TensorFlow, dramatically speed the delivery of AI-enriched insights by making it faster to:
- Blend and organize a data pipeline that AI-models can consume
- Create AI models using data flow graphs and more advanced algorithms
- Train models using advanced parallel architectures and GPUs
- Plug models back into data pipelines so analysts can derive new insights
- Operationalize the AI-enriched pipelines at scale with confident governance
It is not simply a matter of a closer workflow. A recent test using a standard Generalized Linear Model showed that TensorFlow models could be trained 4 times faster than Spark, and 100 times faster than R.
2. Create better models
Feeding more data to any AI model produces greater accuracy and better results. This is especially true for convolutional neural network models, which continuously learn as more data is fed through it.
Combining big data pipelines from your data lake with AI models can pump more data through the models to deliver the desired increase in accuracy. It also allows you to take advantage of data gravity, running models directly on your data lake.
By way of example, one Datameer customer expanded the amount of data they analyzed for fraud detection from 6 months to 3 years. This produced far more accurate results and identified more fraud patterns than had been previously detected.
3. Faster IT-confident Operationalization
The highly custom re-implementation process not only slows the delivery of AI-enriched insights to the business owners, but it also flies in the face of the IT team requirements, who desire security, governance and manageability.
The ability to take AI models and plug them directly into analytic data pipelines directly on your data lake facilitates faster deployment AND gives the IT teams the desired execution frameworks that:
- Ensures the data is highly secure and governed
- Allows lineage tracking and auditing to ensure regulatory compliance
- Enables IT teams to manage jobs and performance
The IT teams become partners with the data scientists and business analysts to operationalize AI-enriched insights to the business.
4. Democratization of data science work
Data scientists do tremendous work that often goes unnoticed. Bringing together a big data engineering platform with an AI platform enables data scientists to share their models with everyday business analysts who apply them to business processes.
Data scientists can share models with business analysts through TensorFlow. From there business analysts can import models into their Datameer workflows and generate AI-enriched insights that can feed everyday business processes.
Is the AI revolution ready to take off?
Taking AI models and insights data scientists have created from the labs to the business is an important step. Bringing together the big data engineering and AI platforms not only helps create faster cycles and easier operationalization, but it also unites data scientists, business analysts and IT into a common team to deliver better results from AI to the business.