Achieving Excellent Data Quality With Datameer
- Jeffrey Agadumo
- May 30, 2023
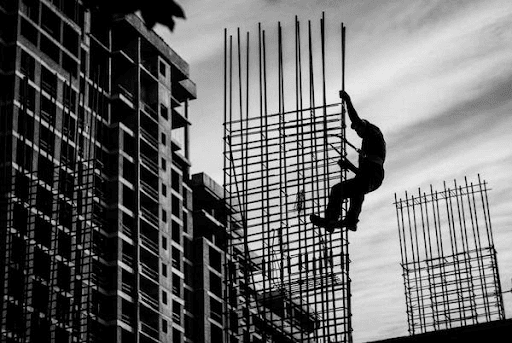
Welcome to the exciting world of data quality, where every byte has the potential to revolutionize your business and elevate you to new heights of success.
Clean and accurate data sets industry leaders apart in today’s data-driven world, but achieving top-notch data is no easy task – it requires commitment, strategy, and finesse.
Are you ready to dive into data quality and unlock the key to making your organization’s data more accurate, consistent, and valuable? Join us on an exciting journey as we demystify data integrity and show you the incredible impact of exceptional data quality on your analytics and decision-making capabilities in this article.
Together, we’ll explore the captivating dimensions of data quality, reveal some of the causes and pitfalls of poor data quality, and unveil the best practices that will elevate your data to new heights. So, let’s get started and unleash the true potential of your data—your organization’s greatest asset!
What is Data Quality?
Good data quality is a sturdy foundation for an organization’s decision-making, insights, and overall performance. It provides the necessary support for growth, adaptability in the face of challenges, and improved competitiveness. Conversely, a weak foundation constructed with inferior materials can result in misguided decisions, operational inefficiencies, and reduced competitive edge.
Data personnel is vital in maintaining and improving data quality, similar to architects, engineers, and construction workers in building a solid foundation. They must use the right tools, best practices, and strategies to ensure the organization’s data foundation remains strong and reliable.
Data quality is a measure of how trustworthy and reliable data is for making informed decisions. It refers to the degree to which data meets specific dimensions, ensuring it produces the best possible results. In other words, data quality is all about trusting the data you’re using to make informed decisions.
Dimensions of Data Quality: Keys to a Solid Data Structure
Data quality dimensions form the crucial components of a solid data foundation, enhancing a dataset’s overall integrity. By addressing these key dimensions, organizations can bolster the value and reliability of their data :
- Accuracy: The extent to which data correctly represents the real-world entities or events intended to describe, removing all errors and inaccuracies.
- Completeness: The degree to which all required data is available and not missing. Complete data ensures that all necessary information is present for analysis and decision-making.
- Consistency: Uniform data across sources, systems, and periods ensures consistency, reliability, and confidence in analysis and decision-making. Consistent data follows the same format, conventions, and rules, making it easier to combine and compare.
- Timeliness: The availability of data when needed and its relevance to the current time, enabling organizations to make well-informed decisions based on the most recent information.
- Uniqueness: Uniqueness ensures that there are no duplicate records in a dataset, eliminating redundancy and ensuring the distinctness and meaningfulness of each data entry.
- Validity: The degree to which data conforms to predefined rules, formats, or standards. Valid data adheres to established data models, schemas, or business rules.
- Relevance: The extent to which data is applicable and valuable for the intended purpose or context. Relevant data aligns with the needs and goals of the organization.
Poor Data Quality: Causes and Pitfalls
A Gartner publication states that 40% of business initiatives fail to reach their objectives due to inadequate data quality, highlighting the harmful impact of poor-quality data on an organization’s progress.
So how does a business end up with poor-quality data anyway?
- Human error: Data is often collected, entered, and processed by human beings who may make mistakes or overlook important details. These errors include typos, incorrect data entry, or failure to verify data accuracy.
- Incomplete data: Data may be incomplete if certain data elements are missing or not collected, resulting in gaps in analysis or inaccurate conclusions.
- Data integration issues: Integrating data collected from multiple sources can be challenging, often leading to inconsistencies or duplication.
- Outdated data: Over time, data can become outdated, failing to reflect the current state of the object or phenomenon it represents. As a result, this inaccuracy in analysis or drawing incorrect conclusions may occur.
- Data bias: Data bias may occur when data is collected or analyzed in a way influenced by the researcher’s beliefs or perspectives, resulting in skewed analysis or inaccurate conclusions.
Dependence on low-quality data for gaining insights and making decisions in daily business operations can lead to organizational pitfalls such as:
- Inaccurate analysis: Poor data quality can lead to inaccurate analysis, resulting in incorrect conclusions and bad decisions.
- Wasted resources: Poor data quality often necessitates organizations to invest additional time and resources in cleaning and correcting the data before utilizing it for analysis.
- Reduced productivity: Poor data quality can lead to delays in decision-making processes and can slow down operations.
Data Quality Best Practices: Big Data and Beyond
An organization that is well aware of these pitfalls ought to do its due diligence to ensure the quality of its data. To that end, it should adhere to some data quality best practices that guarantee the integrity of its data.
It goes without saying that the larger the data set, the more attention you will need to put into data cleaning and preparation before use. As big data becomes increasingly popular amongst businesses and researchers alike, the need arises to have measures in place to measure and track data quality, no matter the size of your data.
So what does due diligence entail for ensuring data quality?
1. Establish Data Quality Standards
Define and document data quality standards that outline the specific requirements for data accuracy, completeness, consistency, timeliness, validity, and reliability. Establishing clear data quality standards can help ensure everyone in the organization is on the same page and adhering to best practices.
2. Conduct Data Profiling
Data profiling analyzes data to identify potential issues, such as inconsistencies or missing values. Regular data profiling can help identify and address data quality issues before they become major problems.
3. Implement Data Validation Processes
Implementing data validation processes is essential to ensure data accuracy, completeness, and consistency. These processes can involve automated or manual validation methods.
4. Perform Data Cleansing
Data cleansing is identifying and correcting data errors, inconsistencies, and inaccuracies. Regular data cleansing can help ensure that data is accurate, complete, and consistent.
5. Ensure Data Security
Protecting data is critical to maintaining data quality. Ensure that data is secure by implementing appropriate access controls, encrypting sensitive data, and regularly monitoring access to data.
6. Educate and Train Users
Educate and train users on data quality best practices, including collecting, entering, and processing data accurately and consistently. This practice helps ensure that everyone in the organization is contributing to high-quality data.
7. Implement Data Governance
Implement a data governance framework to establish policies and procedures for managing data quality. Incorporating governance involves assigning responsibility for data quality to specific individuals or teams and conducting regular reviews of data quality metrics to ensure adherence to standards.
Ensuring Top-Notch Data Quality with Datameer and Snowflake
Analyzing and validating data has become increasingly challenging as businesses experience a growing volume of data, including unstructured data such as social media posts, videos, and images. As a result, many forward-thinking businesses are turning to cloud storage solutions to overcome this challenge. One such popular solution is Snowflake, which provides advanced data warehousing capabilities in a modern data stack.
You can automatically access Datameer and all its associated features by choosing Snowflake as your cloud storage provider. Together, Snowflake and Datameer make a powerful duo in ensuring the quality of an organization’s data. Here are some examples of how Snowflake and Datameer can collaborate to ensure data success:
- Dynamic Data Integration
Datameer simplifies data integration with more than 70 connectors to common enterprise data sources and an SDK and REST API. The wizard-led integration process requires no schemas or advanced modeling, enabling fast integration of raw data from various sources, including Snowflake. In addition, Datameer’s schema-less architecture facilitates live data access without pre-loading or creating a separate copy of data. Instead, users can upload files, import datasets, or use data links to generate an instantly available, virtualized view.
- Visual Data Profiling
Datameer’s data profiling features, including visual data profiling, system-generated recommendations using machine learning, and system- and user-generated data profile information, facilitate data discovery through faceted search and allow users to explore datasets and data models that meet certain profiles. In addition, with full integration with Snowflake, Datameer’s data profiling tools offer further context and profile information on the data, enabling users to identify preparation needs and take actions to optimize data quality.
- Intuitive Data Governance
Datameer’s governance features seamlessly integrate with enterprise and cloud security, offering asset-level controls and encryption at rest and in transit. In addition, it fully integrates with Snowflake security. It offers multiple forms of metadata, user-defined properties, standardized tags, and AI to detect Personally Identifiable Information (PII), enabling specific handling controls. Datameer’s governance also includes a complete audit trail of all aspects, including details on data usage, making it an ideal solution for Snowflake users looking for robust governance.
At Datameer, data quality isn’t just a buzzword – it’s their top priority. With its innovative technology and rigorous quality control measures, you can trust that your data is in the best hands possible.
So my advice? Don’t settle.
Partner with the data experts – Datameer and Snowflake – and let us help you unlock the true potential of your data and drive your business forward!