Six Steps for Answering Ad Hoc Business Questions with Analytics
- Benoite Yver
- February 25, 2020
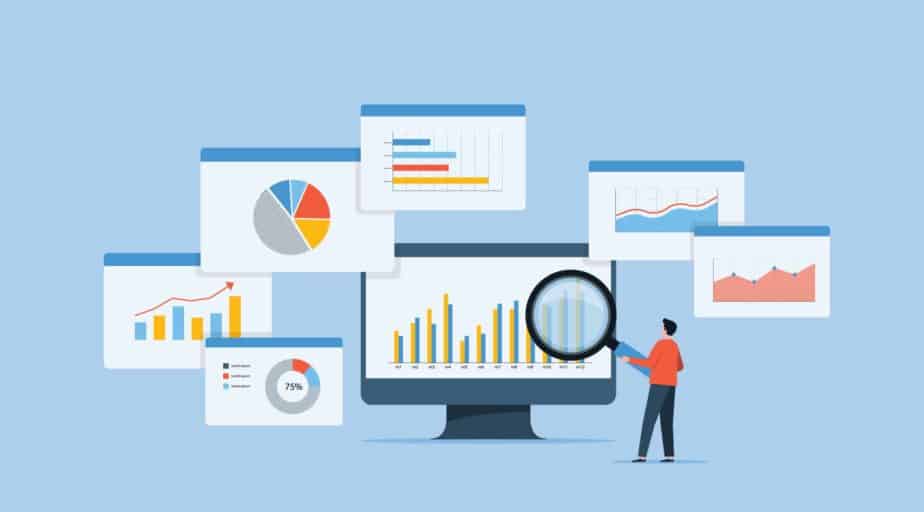
By strategically approaching ad hoc data analysis, you’ll be able to leverage the information, software, and services at your disposal to transform raw data into valuable insights for intelligent decision making.
Big data and analytics are critical in today’s competitive landscape. They deliver insights that guide organizations to make better business decisions, boost revenue through streamlining operations, track performance, and improve customer experience.
6 Step Ad Hoc Analysis
Here are six steps analysts can follow to answer ad hoc business questions for data-driven answers:
1. Quantify the business question
Business questions are often vague or not fully defined from an analytics perspective. Take, for example, a question from an e-commerce retailer’s CMO – “Who are our best customers? How do we acquire more of them?”. It feels like a simple business question at first, but it isn’t fully defined. Let’s start breaking this down. What do we mean by “best customers”? It could mean several things from High Lifetime Value or Low Cost Per Acquisition to something completely non-monetary such as customers that engage most with our social content and are great influencers and brand builders for us. How we define “best customers” will influence how we answer the question about acquiring more of them.
Before starting your analysis, come up with quantifiable definitions for the questions at hand and get alignment from the business on whether that’s the right/appropriate quantified interpretation of the item. Asking for the context behind the problem will also help you develop possible quantifiable definitions of the question.
2. Generate Hypotheses
Upon defining your specific business question, the common pitfall is to start “boiling the ocean,” gather data you have, get access to the data you don’t have and might need, and grind that into the hottest data science model in vogue. Even though that approach might get you to find some useful insights, it’s neither the most scientific nor the most economical way to solve a problem. Use your domain expertise to generate hypotheses. Say the quantified business question is, “How do we increase revenue by 10% a year?”. You could hypothesize that it can be done by hiring more salespeople, spending more on marketing, doing more cross-selling or upselling, expanding into new markets, and so on.
3. Determine the Analysis method
Once you have some hypotheses, you can select one or more analytical methods to test these hypotheses. Think about the desired output and actionability of it. Analytical methods could include any of the following and more,
- Predictive models
- A/B testing
- Time Series analysis
- Machine Learning techniques such as clustering, classification, or NLP
During this phase, you will also want to answer questions about the data and metrics you will need for the analysis. A few questions to answer would be,
- What are the different types of data that I need?
- What are the features I need in that data?
- How much data do I need to apply the desired analytical method?
- Are there any derived metrics and calculations I need to perform?
Being clear on these questions will help you validate your chosen method and adjust it promptly as you start discovering and exploring the available data. For instance, if a specific type of information isn’t available, or if you don’t have enough historical data about something, the chosen analytical method may turn out to be ineffective.
4. Data Discovery and Transformation
With your ad hoc business question quantified, hypotheses generated, and the analytical method proposed, it is time to discover, collect, organize, and prepare your data.
Start by the discovery of existing data sources within your organization. Explore where information is stored – within databases, in the cloud, or SaaS applications. You may have to tackle pain points before discovering and exploring all the data you need. e.g., your data sits in silos, you don’t know if the company even has specific data that you are looking for, and you don’t know who to talk to about it, etc. It may take several days to discover and get access to all the data you need before you can start exploring it.
Once you’ve located and gained access to all the data you need, you will want to figure out the best way to start exploring and preparing the data for your analysis. Depending on how centralized all the data is and what BI or Data Science tools it is accessible from, you may start working with it right away. Maybe you will have to go to the lengths of creating extracts from multiple systems and stitching everything together offline in excel or another tool.
In most organizations, depending on the degree of data silos and collaboration between teams, iterations between step 3 (Determine the Analysis method) and stage 4 (Data Discovery and Preparation) can often consume a significant portion of your overall turnaround time for answering the business question.
5. Perform the Analysis
At this stage, you’re ready to take your final dataset and analyze it so you can start looking for answers to your business question. How you’ll do this will depend on the analysis method chosen and the relevant tools you have accessed. You may want to use a business intelligence (BI) tool such as Tableau or Looker or a Data Science tool such as Jupyter Notebook for more in-depth statistical analysis, or both. Your ability to seamlessly connect your final dataset to multiple devices will drive efficiency and reduce time to insights for agile data-driven decision making.
As you interpret data — or if the analysis hits a dead end — you may discover that you need to reconsider your earlier steps. You may need to rethink how data was collected and prepared, if any key factors were overlooked, or even revisit your original framework’s applicability to this specific business question.
Once again, the agility with which you can iterate between step 3 to step 5 will be driven by the degree of data silos, ability to collaborate, and access to tools to work with the data.
6. Share Findings and Make Recommendations
Well done! If your data analysis holds up to the questions and hypotheses put forth in the earlier steps, you’re getting close to sharing your findings with business decision-makers. It’s now time to find a way to represent your findings in a consumable format.
If you are using a BI tool, you’ll have the ability to create dashboards with sophisticated visualization options that are easy-to-use and share. If not, you may need to make your visualizations such as charts, graphs, maps, or other graphics in a presentation tool like Microsoft PowerPoint or Google Slides.
Regardless of what tool you use, it is critical to keep in mind the audience for your report so that you can provide relevant context and an appropriate level of detail before making your recommendation. Depending on the organization’s role and status, you may need to make tradeoffs between your analysis’s technical depth and the strategic implications of the results and recommendations. While you may want to share specific details about the models, data prep, etc., the leader of your analytics team, you may wish to only abstract out the key findings, implications, and recommendations for the business decision-maker. Always keep in mind who you are presenting to and what is it that matters most to them.
Bringing it all together
While it is essential to answer business questions with data in the most comprehensive manner possible with actionable results and recommendations, it is just as or even more important to do so with extreme agility in today’s fast-changing business world. However, that is often not the case. Analytics professionals spend way too much time trying to find, access and prepare the data they need for the analysis while working within data and organizational silos and in the absence of proper analytics knowledge management and collaboration. We haven’t talked about analytics knowledge management in this article, but you can read about it in-depth in our post about why analytics knowledge management matters .
Datameer SaaS Data Transformation is the industry’s first collaborative, multi-persona data transformation platform integrated into Snowflake. The multi-persona UI, with no-code, low-code, and code (SQL) tools, brings together your entire team – data engineers, analytics engineers, analysts, and data scientists – on a single platform to collaboratively transform and model data. Catalog-like data documentation and knowledge sharing facilitate trust in the data and crowd-sourced data governance. Direct integration into Snowflake keeps data secure and lowers costs by leveraging Snowflake’s scalable compute and storage.
Learn more about our innovative platform, Sign up for your free trial today!